Invited Speakers 特邀嘉宾
Prof. Lei Cheng
Zhejiang University, China
Bio: Lei Cheng is currently ZJU Young Professor with the
College of Information Science and Electronic
Engineering, Zhejiang University, Hangzhou, China.
He received the B.Eng. degree from Zhejiang
University in 2013, and the Ph.D. degree from The
University of Hong Kong in 2018. He was a Research
Scientist in Shenzhen Research Institute of Big
Data, The Chinese University of Hong Kong, Shenzhen,
from 2018 to 2021. He is the author of the book
“Bayesian Tensor Decomposition for Signal Processing
and Machine Learning”, Springer, 2023. He was a
Tutorial Speaker in IEEE ICASSP 2023, Invited
Speaker in ASA 2024 and IEEE COA 2014. He is now
Associate Editor for Elsevier Signal Processing
and Young Editor for Acta Acustica.
Prof.
Jun Liu
Chengdu University of Information
Technology, China
Bio: Jun Liu received the Ph.D. degree in
control science and engineering from the University
of Electronic Science and Technology of
China(UESTC), Chengdu, China, in 2018. He is
currently a Professor with the School of Automation,
Chengdu University of Information Technology (CUIT),
Chengdu, China. In 2014, he worked with the
University of California at San Diego (UCSD), San
Diego, CA, USA, as a Visiting Scholar. From 2020 to
2021, he worked with the Hong Kong Polytechnic
University (PolyU), Hong Kong SAR, China, as a
Postdoctoral Fellow. He has authored or coauthored
more than 60 articles in various journals and
conference proceedings, and has won several
provincial and ministerial awards. He has served as
the Sichuan chairman in China of the 5th, 6th and
7th Asian International Conference on Artificial
Intelligence Technology (2021-2023ACAIT), member of
the academic committee of the 7th IEEE International
Conference on Data Science and Systems (IEEE
DSS-2021), member of the technical procedure
committee and co-chairman of the organization
committee of the 14th, 15th and 16th International
Conference on Signal Processing Systems
(2022-2025ICSPS), member of the organization
committee of the 2nd International Conference on
Robotics, Artificial Intelligence and Intelligent
Control (2023RAIIC), member of the procedure
committee of the 30th International Conference on
Neural Information Processing (ICONIP2023), etc. He
has served as served as a special reviewer of IEEE
Transactions on Industrial Information, IEEE
Transactions on Neural Networks and Learning
Systems, IEEE Transactions on Cybernetics, ISA
Transactions, Neurocomputing, Journal of the
Franklin Institute, Journal of Engineering Science
(CJE), Journal of Intelligent Systems and other
journals, as well as editorial board members of some
journals. He has served as a commentator of
Mathematic Reviews in the United States. His
research interests include coordinated control of
multi-agent systems, machine learning, new computing
and its applications, artificial intelligence and
robots.
Assoc. Prof. Guobing Qian
Southwest University, China
Bio: Guobing Qian is a Senior Member of both the IEEE and
the Chinese Institute of Electronics. He received
the Ph.D. degree in signal and information
processing from the University of Electronic Science
and Technology of China in December, 2015.
Currently, he serves as an Associate Professor at
the College of Electronic and Information
Engineering, Southwest University. His primary
research interests encompass adaptive filtering and
adaptive signal processing.
He has been invited to serve as a Guest Editor for
special issues in SCI-indexed journals, including
Electronics and Symmetry. Since 2022, he has
consecutively served as the Co-Chair of the Program
Committee for the International Conference on
Digital Signal Processing (ICDSP) from its 6th to
9th sessions. Furthermore, he has been appointed as
the Co-Chair of Publications for the 6th
International Conference on Electronic Engineering
and Informatics (EEI) in 2024.
Assoc. Prof. Fang Yang
Wuhan University of Science and Technology, China
Bio: Dr. Fang Yang is an associate professor at Wuhan
University of Science and Technology and a recipient
of the Hubei Province "Chutian Young Scholar" title,
with primary research interests in multi-modal
remote sensing image fusion and geodesic methods.
Her work focuses on advancing techniques for
denoising, image registration, and change detection,
aiming to improve the analysis and interpretation of
remote sensing data. Dr. Yang is the principal
investigator of a National Natural Science
Foundation Youth Project and has been involved in
several other National Natural Science Foundation
projects. With over 30 publications in international
journals and conferences, her research contributes
to the development of innovative methodologies for
remote sensing applications. Through her work, she
seeks to integrate cutting-edge mathematical models
and computational methods to address key challenges
in image processing.
Assoc. Prof. Zipeng Li
Northwestern Polytechnical University, China
Bio: Zipeng Li is an Associate Professor and Master’s
Supervisor at the School of Marine Science and
Technology, Northwestern Polytechnical University
(NPU). He holds a Ph.D. degree and has been engaged
in long-term research on signal processing theory
and underwater acoustic signal processing
technologies. His work focuses on strong-noise
non-stationary signal processing, weak signal
feature extraction, and deep learning-based
underwater target classification.
Li began his academic journey in 2010 at Xi’an
Jiaotong University (XJTU), where he studied in the
School of Mechanical Engineering. He graduated with
a double bachelor’s degree in 2014 and was directly
admitted to a Ph.D. program. From 2017 to 2019, he
conducted joint Ph.D. training at the University of
Toronto, Canada, under the supervision of Prof.
Viliam Makis, a renowned expert in operations
research and signal processing. He obtained his
Ph.D. in Mechanical Engineering from XJTU in 2020.
His primary research directions include:
•Signal processing theories and innovative methodologies
•Active/passive sonar underwater detection
•Underwater acoustic target classification and recognition
Research interests encompass:
•Novel adaptive signal processing methods applied to
acoustic/vibration signals
•Extraction of extremely weak features from high-noise
non-stationary signals
•Deep learning-driven underwater target classification techniques
Assoc. Prof. Xiaoying Song
Wuhan University of Science and Technology, China
Bio: Xiaoying Song received the B.S. degree in electronic
science and technology and the Ph.D. degree in
microelectronics and solid-state electronics from
Wuhan University, Wuhan, China, in 2012 and 2017,
respectively. Since July 2017, she has joined the
School of Information Science and Engineering at
Wuhan University of Science and Technology, Wuhan,
where she was recognized as a Chutian Scholar of
Hubei Province. Her research interests include graph
signal processing, graph learning, and their
applications in brain network analysis,as well as
QSAR/QSPR model learning of compounds.
Assoc. Prof. Guchong Li
Northwestern Polytechnical University, China
Bio: Guchong Li received the B.S. degree in electrical
engineering and Ph.D degree in information and
communication engineering from the University of
Electronic Science and Technology of China (UESTC),
Chengdu, China, in 2016 and 2021, respectively. From
October 2018 to October 2020, he was a Visiting
Student at the University of Florence, Italy. From
July 2021 to July 2023, he was a Postdoctoral Fellow
with the Department of Electronic Engineering,
Tsinghua University, Beijing, China. He is currently
an Associate Professor with Northwestern
Polytechnical University. His current research
interests include random finite set, target
tracking, and multi-sensor data fusion.
Assoc. Prof. Hui Tang
Wuhan University of Science and Technology, China
Bio: Hui Tang received the B.E. degree in electrical and
electronic engineering and the Ph.D. degree in radio
physics from Wuhan University, China, in 2011 and
2016, respectively. She was a visiting Ph.D. student
at Arizona State University, USA, from September
2014 to August 2016. She is currently an Associate
Professor at Wuhan University of Science and
Technology. Her research interests include machine
learning, graph neural networks, and radar signal
processing.
Assoc. Prof. Wei
Wang
National University of Defense Technology, China
Bio: Wang Wei received the M.S. degree in information and
communication engineering from National University
of Defense Technology, China, and the Ph.D. degree
in geoinformatics from KTH Royal Institute of
Technology, Sweden, in 2013, and 2018, respectively.
He is currently an associate professor with
Automatic Target Recognition (ATR) National Key
Laboratory, National University of Defense
Technology. His research interests include
SAR/PolSAR image processing, automation target
recognition, target characteristics and data
engineering. He has published more than 60 journal
and conference articles, and obtained two
provincial-level scientific research awards. He was
recognized as Young Talent of Hunan Province in
2023. He is an editorial board member of Aero
Weaponry, and acts as a guest editor of Electronics.
He is also a reviewer of high-level international
journals, such as IEEE Transactions on Geoscience
and Remote Sensing, IEEE Transactions on Intelligent
Transportation Systems, and Pattern Recognition.
Assoc. Prof. Chang Liu
Dongguan University of Technology, China
Bio: Chang Liu, D.Eng, Associate Professor, he received
the Ph. D. degree in the School of Electronic
Engineering, UESTC, Chengdu, P. R. China in 2012.
From 2012 to 2014, he was with Huawei Technologies
Company Ltd., Chengdu, China, as a radio frequency
algorithm engineer, working on the anti-interference
technologies at intermediate and radio frequencies.
Since 2014, He has been with School of Electronic
Engineering, Dongguan University of Technology,
Dongguan, China, where he became an Associate
Professor in 2019. His current research interests
include adaptive signal processing, array signal
processing and interference cancellation in the
fields of audio and radio frequency.
Assoc. Prof. Xiaosi Tan
Southeast University, China
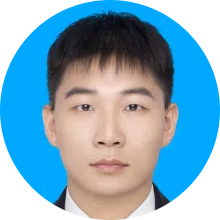
Harbin Engineering University, China
Bio: Xu Chen received the M.S. degree from Northeastern University (NEU), Shenyang, China, in 2020 and the Ph.D. degree from National University of Defense Technology (NUDT), Changsha, China, in 2024. He is currently an Associate Professor at College of Information and Communication Engineering, Harbin Engineering University (HEU), Harbin, China. His research interests include millimeter-wave radar imaging, MIMO array signal processing, and radar electronic countermeasure technology. As a key team member, he has participated in multiple projects, such as the National Key R&D Program, the National Natural Science Foundation, and the National Defense Technology Plan. To date, he has authored over ten SCI-indexed journal papers (one of which ranks in the top 1% of ESI highly cited papers for 2023). He is a long-term reviewer for high-level SCI journals such as IEEE Transactions on Instrumentation and Measurement (TIM), IEEE Transactions on Computational Imaging (TCI), IEEE Transactions on Microwave Theory and Techniques (TMTT). In 2025, he received the Outstanding Reviewer Award from IEEE TIM, a top journal in the field of instrumentation and measurement.
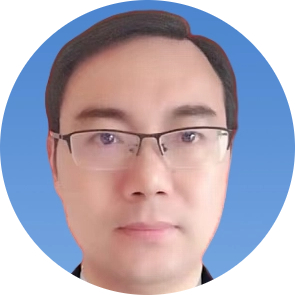
Guizhou University, China
Bio: Zhang Yongjun (IEEE Member), PhD, Master's Supervisor/Associate Professor, Visiting Scholar at Cardiff University, UK, Executive Dean of the Artificial Intelligence Research Institute of Guizhou University, Director of Guizhou Provincial Remote Sensing Big Data Innovation Center. Council Member of the National Computer Education Research Association of Institutions of Higher Learning, Level 2 Innovation Method Instructor in Guizhou Province, Member of the Chinese Association of Artificial Intelligence, Member of the Expert Consultation Committee of the China Security Protection Industry Association, He has published over 50 research papers in computer vision at top computer conferences/journals such as CVPR, ACCV, and IEEE Transactions, and has applied for 15 invention patents. His research focuses on intelligent video analysis and image processing, including binocular stereo matching, object detection and recognition, image enhancement, remote sensing image processing, and engineering applications,etc.
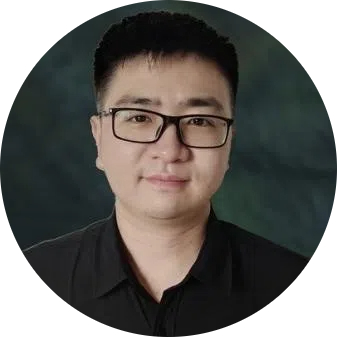
The Hong Kong Polytechnic University, Hong Kong, China
Bio: Ran Duan received a B.S. degree in Communication Engineering from the School of Information Engineering, Southwest University of Science and Technology in 2013 and a Master's degree in Computer Vision and Robotics from the University of Burgundy, France (European VIBOT program) in 2015. From 2015 to 2017, he worked as a research associate at Nanyang Technological University (NTU), Singapore. In 2022, he earned his Ph.D. degree from the Department of Aeronautical and Aviation Engineering (AAE) at the Hong Kong Polytechnic University. He is currently a Research Assistant Professor in the Department of Land Surveying and Geo-Informatics at the Hong Kong Polytechnic University. His recent research interests mainly include visual positioning and navigation of unmanned aerial vehicles (UAVs), visual odometry, VSLAM, target Technical Symposiaing, etc. He has authored several top conference and journal papers in the field of intelligent robots and smart vision, including IROS, ICRA, EAAI, IEEE TMECH, IEEE TIM, IEEE JSTARS etc. He has supported or participated in a number of scientific research projects funded by the Dijon Agricultural Sciences Institute in France, Strasbourg University Hospital in France, Singapore Technologies Engineering Limited, and other institutions. Dr. Duan has long served as a reviewer for the conferences IROS and ICRA in the field of intelligent robots. He won the third place in the autonomous drone racing competition at the 2019 IROS conference and became one of the committee members of the Remote Sensing Surveying and Mapping for Deep Space Exploration, Chinese Society for Geodesy Photogrammetry and Cartography in 2022.
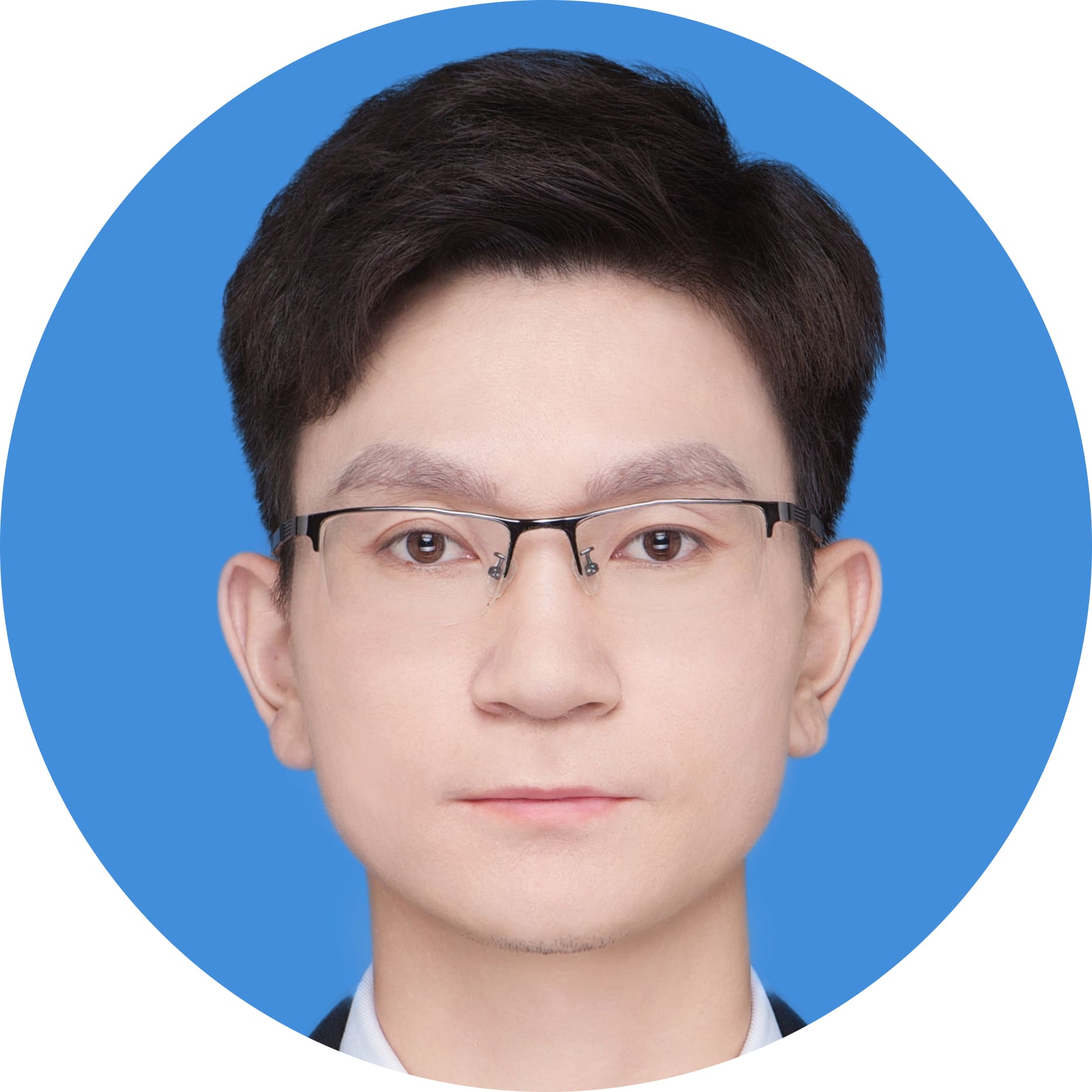
Chang’an University, China
Bio: Dr. Zuo Luo is currently a Lecturer at Chang’an University. He received his Ph.D. in Signal and Information Processing from Xidian University in 2022, under the supervision of Prof. Jun Wang. His research focuses on BeiDou and internet satellite signal analysis, passive radar system design, and weak target detection. In 2023, Dr. Luo joined Xidian University as a Postdoctoral Researcher, working with Prof. Congsi Wang.
He has actively participated in several national-level research projects, including the National Natural Science Foundation of China (NSFC) - Youth Program and the Guangdong Natural Science Foundation - General Program (2025). As a prolific researcher, Dr. Luo has published over 10 first-author/corresponding-author papers in prestigious international journals such as IEEE TAES, IEEE TGRS, DSP, and IET RSN.